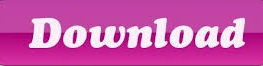
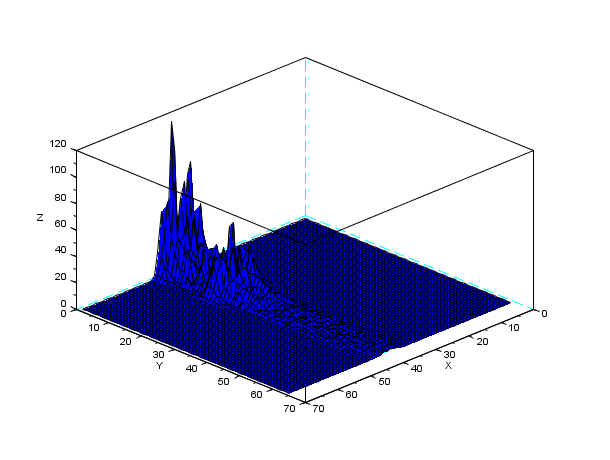
This was not observed in the circle due to the circle being symmetric.Īside from circles and letter A, I also applied FFT to other patterns. Applying the FFT twice results in an inverted A. Similar to the circle, we see how the fftshift()function corrects the positioning of the quadrants after FFT. imwrite(uint8(abs()), "FFT_LetterA.png")įigure 2. Letter A, the unshifted FFT result, the shifted FFT result, and result of using FFT twice.įigure 2 shows the original letter A image, the unshifted FFT result, the shifted FFT result, and the result of using FFT twice. Imshow(uint8(abs())) //converts to 8-bit unsigned integers Take the FFT twice for the original imageįftdouble_A = ((255*abs(fftdouble_A))/max(abs(fftdouble_A))) Convert the arrays corresponding to the circles to "double" and take the FFT of the loaded imageįft_A = ((255*abs(fft_A))/max(abs(fft_A))) įftshift_A = ((255*fftshift_A)/max(fftshift_A)) Load a 128 x 128 px image of letter A with a black background
#Scilab fft example code
The code used to process the letter A is shown below: We apply the same steps followed in the circle to a letter A typed on a black background in Paint. Based on how FFT shifts the quadrants, the circles are actually inverted but it is not apparent because of the circle’s symmetry. Applying the FFT twice on the circle, we can see that we get the original image. Anamorphism is also observed here, as increasing the radius of the circle decreases the size of the Airy pattern in Fourier space. The fftshift()function corrects the positions of the quadrants that are rearranged after the FFT. We can see that the Fourier transform of a circle is an Airy pattern. The result upon applying fft2()twice was also tested.įigure 1. Circles of increasing radii, the unshifted FFT results, the shifted FFT results, and result of using FFT twice.įigure 1 shows the original circle images, the unshifted FFT result, the shifted FFT result, and the result of using FFT twice. The FFT results are then shifted using fftshift()for the results to be ready for displaying. the fast Fourier transform of the three circles are taken, and are normalized to be displayed by the imshow()function. imshow(uint8()) //converts to 8-bit unsigned integersĪs seen in the code, three images of circles are loaded. Combines arrays side-by-side for displayingįft_multi1 = įft_multi2 = įftdouble_circle1 = fft2(fft2(double(circle1)))įftdouble_circle2 = fft2(fft2(double(circle2)))įftdouble_circle3 = fft2(fft2(double(circle3)))įftdouble_circle1 = ((255*abs(fftdouble_circle1))/max(abs(fftdouble_circle1))) įftdouble_circle2 = ((255*abs(fftdouble_circle2))/max(abs(fftdouble_circle2))) įftdouble_circle3 = ((255*abs(fftdouble_circle3))/max(abs(fftdouble_circle3))) įftdouble_multi1 = Take the FFT shift of the original FFT and do the same normalization to 8-bitįftshift_circle1 = fftshift(abs(fft_circle1)) įftshift_circle2 = fftshift(abs(fft_circle2)) įftshift_circle3 = fftshift(abs(fft_circle3)) Convert the arrays corresponding to the circles to "double" and take the FFT of the loaded circle imagesįft_circle1 = ((255*abs(fft_circle1))/max(abs(fft_circle1))) įft_circle2 = ((255*abs(fft_circle2))/max(abs(fft_circle2))) įft_circle3 = ((255*abs(fft_circle3))/max(abs(fft_circle3))) Load three different sets of circles of varying radii. The 2D Fast Fourier Transform of Scilab was tested first on 128 x 128 monochrome images of circles of different radii. To display the FFT, fftshift() is used to rearrange the array for displaying and the absolute value of the complex array is taken using abs(). These functions are most efficient when the data processed as a size of the power 2 and results in a complex array. In Scilab, the FFT functions are fft()and fft2()for 1D and 2D signals, respectively. For images with space as its physical dimension, the Fourier transform is in spatial frequency. The Fourier transform is a linear transform that transform a physical dimension to its inverse. I used Scilab 5.5.2 with the SIVP package. In this blog post, I’ll explore the Fourier transform and its use in image processing. Back then, I thought that was pretty cool. Pure tones of frequencies corresponding to the peaks in the FT are combined to produce the sound. For example, we simulated the sound of a musical instrument by sampling the sound of the aforementioned instrument and taking the Fourier transform of the sample sound. During these classes, we mostly used the Fourier transform for processing sound signals. I first encountered the Fourier transform in my Applied Physics 183 and Applied Physics 185 classes. Exploring the Fourier transform in imaging processing
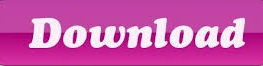